Accurate demand forecasts help companies plan production, control stock, and keep customers happy. Traditional methods rely on past sales and manual patterns that break down when markets change quickly. AI in demand forecasting actually works with the usage of machine learning which helps it to easily analyze the large datasets that are being fed then it aims to spot patterns and update the predictions all in real time.
By applying AI forecasting, businesses can reduce stock gaps, cut waste, and respond faster to shifts in customer needs. As AI based demand forecasting grows, leading companies gain a competitive edge through clear insights and reliable guidance. This blog explains why top firms adopt AI for demand forecasting and how they benefit.
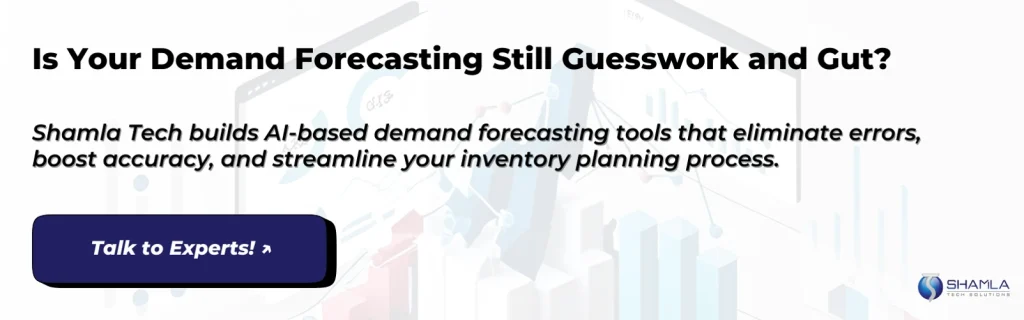
What Is AI in Demand Forecasting?
AI in demand forecasting means using computers that learn from data to predict what customers will buy. Instead of relying on simple averages or fixed rules, AI based demand forecasting systems chew through sales numbers, web visits, promotions, and external factors like weather or holiday trends. These systems spot hidden links in the data that humans can miss. They keep learning and improving as new information comes in.
Traditional methods often use spreadsheets and fixed formulas. They look back at last year’s numbers and apply a percentage increase or decrease. That fails when a sudden event or trend changes buying habits. AI forecasting can handle many data streams at once and adapt its models right away.
Key features:
- Real‑time updates: AI adjusts forecasts as new sales or web data arrive.
- Self‑learning models: The more data fed in, the smarter the predictions.
By moving to AI based demand forecasting, companies leave behind rigid, slow methods. They gain a tool that sees patterns fast and acts on them. This leads to tighter stock control, fewer missed sales, and lower holding costs.
Why Traditional Forecasting Methods Are Failing?
Many firms still use old tools that run on fixed formulas and manual entry. These tools have three big problems:
- Inaccuracy: They assume the future looks like the past. When trends shift, forecasts go wrong.
- Slow updates: Each time you want a new forecast, someone must export data, set up the sheet, and run formulas. That can take days.
- Data silos: Data related to sales, marketing, website activity, and suppliers is often spread across multiple independent platforms, making it difficult to access and analyze in one place. Manual merging leads to errors.
Manual steps introduce mistakes. A missing formula update or a copy‑paste error skews the forecast. Granularity is low too, teams see only monthly totals, not daily or hourly demand. This makes it hard to spot sudden spikes or dips.
AI for demand forecasting solves these issues. It links up all data sources, runs updates automatically, and delivers precise numbers down to the SKU level. Instead of waiting for weekly reports, teams get alerts when demand shifts. They can act fast on supply orders, pricing moves, or marketing pushes.
When you get your work done with AI based demand forecasting, it helps you in an amazing way which predicts the demand with impeccable data‑driven certainty. They cut manual work, close data gaps, and keep pace with market changes in real time.
Benefits of AI-Based Demand Forecasting for Companies
- Higher Accuracy: AI forecasting reduces error rates by up to 50% compared to manual methods. Its models adjust for seasonality, promotions, and sudden market shifts.
- Faster Predictions: AI systems process millions of data points in minutes. This means daily or even hourly forecasts instead of monthly reports.
- Real‑Time Analysis: AI for demand forecasting pulls in live data, web clicks, social mentions, supplier lead times. It spots changes and updates numbers on the fly.
- Better Inventory Control: Accurate predictions help firms keep just the right amount of stock. They avoid overstock that ties up cash and understock that loses sales.
- Waste Reduction: Food, fashion, and tech companies cut waste when forecasts match real demand. That helps margins and eco‑scores.
- Improved Customer Service: When stock is on hand, shoppers find what they need. That boosts loyalty and repeat sales.
- Scalable Models: AI based demand forecasting scales from a single store to a global network. Adding new products or regions takes minimal setup.
By switching to AI in demand forecasting, companies unlock a range of clear gains. They trade slow, error‑prone steps for quick, data‑backed actions. That frees teams to focus on strategy instead of spreadsheet fixes.
How Leading Companies Are Using AI Forecasting?
Retail: Amazon makes full use of AI forecasting in the prediction of demand across the millions of SKUs. It blends internal sales trends with web search data and local weather. This helps Amazon stock high‑demand items before peaks hit. Walmart runs AI for demand forecasting in its stores to spot local preferences and adjust orders daily.
Manufacturing: Unilever applies AI in demand forecasting to plan production runs for soap, shampoo, and snacks worldwide. Its AI models work in such a way which combines sales, promotion schedules, and even supplier lead times too. That ensures factories run at optimal capacity with minimal delays.
Supply Chain: Maersk, a shipping giant, uses AI forecasting to predict cargo volumes on key routes. By knowing demand in advance, it sets sail with the right container mix and reduces empty runs.
Technology: A cloud hosting firm feeds AI forecasting models with server usage logs. It predicts peak loads and auto‑scales capacity minutes before spikes. This keeps sites online and cuts hosting costs.
Key Takeaways from Major FIrms:
- They integrate AI-driven forecasting with supply chain operations such as procurement, inventory management, and logistics.
- They treat forecasts as living data, not static reports.
- They build feedback loops so actual sales refine future predictions.
These examples show that AI in demand forecasting is not a pilot project, it’s a core tool for top firms. By embedding AI into their planning cycle, they stay ahead of fast‑moving trends and keep costs in check.
Steps to Get Started with AI in Demand Forecasting
- Check Data Readiness: List all data sources: sales, web analytics, promotions, suppliers. Ensure you can access and clean each stream.
- Pick the Right Tools: Choose an AI platform that fits your scale. Look for prebuilt connectors and easy model‑training features.
- Form a Team: Include data analysts, IT, operations, and a project lead. Clear roles speed up setup and cross‑team buy‑in.
- Run a Pilot: Start small with a few SKUs or one region. Compare AI based demand forecasting results to your old method. Adjust models as needed.
- Scale Gradually: Roll out to more products, regions, and channels. Use lessons from the pilot to refine training data and settings.
- Set Feedback Loops: Track forecast accuracy daily. Feed actual sales back into the AI models so they learn and improve.
- Train Staff: Teach teams how to read AI forecasts and act on alerts. Clear processes ensure data insights lead to real decisions.
- Review Regularly: Hold monthly reviews to check accuracy, spot new data sources, and fine‑tune models.
By following these steps, you can introduce AI forecasting smoothly. You move from spreadsheets to live insights with minimal disruption. That sets the stage for real gains in efficiency and revenue.
Challenges to Consider Before Making the Switch
- Implementation Cost: AI platforms and data work carry an upfront price. Be clear on license fees, hosting, and integration costs.
- Data Privacy: Combining internal and external data must follow privacy rules. Check contracts and local laws before blending customer or supplier data.
- Change Resistance: Teams used to manual reports may push back. Show early wins from your pilot to build trust in AI based demand forecasting.
- Skill Gaps: You need data engineers and analysts who understand AI. Plan for training or hire new talent.
- Integration Effort: Connecting sales, marketing, web, and supplier systems can take time. Map out each link and assign owners.
- Model Drift: AI models can lose accuracy over time if not updated. Set a schedule to retrain models with fresh data.
By planning for these risks, you keep your AI for demand forecasting projects on track. A clear budget, strong governance, and ongoing training turn potential roadblocks into manageable tasks.
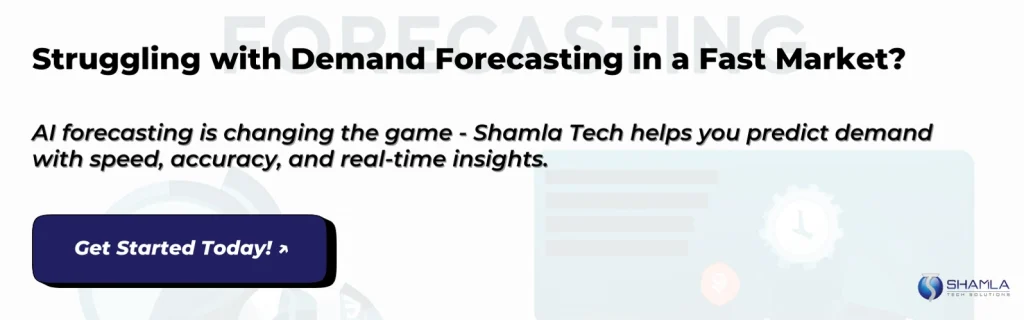
Conclusion
AI in demand forecasting transforms planning from guesswork to clear, data‑driven action. Companies that switch to AI forecasting cut errors, speed up decisions, and lower costs.
Shamla Tech is an AI development company offering solutions for AI based demand forecasting. We’ve helped clients worldwide, from retailers to manufacturers helping them boost forecast accuracy by up to 50% and slash waste by 25%.
By working with Shamla Tech, teams gain tools, training, and support to make AI for demand forecasting a seamless part of operations. If you’re ready to move from spreadsheets to smart predictions, now is the time to adopt AI in demand forecasting.